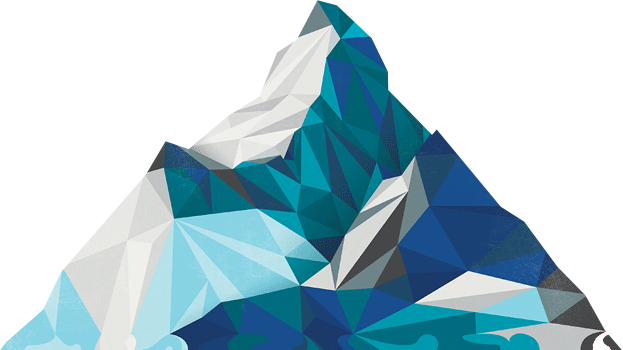
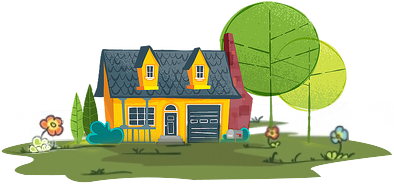

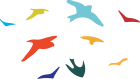

IFM.py is an open-source toolkit released by IFM Lab. The toolkit covers the state-of-art algorithms for information fusion and data mining.
Fusion Learning is a textbook about informaiton fusion and data mining, mainly about the application on heterogeneous social networks.
IFM Lab releases several multi-source datasets on Online Social Networks, Knowledge Graphs, Geographical Locations, Bio-Informatics, etc.
Information Fusion and Data Mining across Multiple Information Sources.
To enjoy different kinds social network services, people nowadays are usually involved in multiple online social networks simultaneously. Fusion and mining multiple aligned online social networks is one of the main stream research project of IFM Lab, which involves research problems like network alignment, cross-network link prediction, community detection, information diffusion, viral marketing, and network embedding etc.
Multiple language systems co-exist simultaneously in the real world, like various natural languages and programming languages. When referring to certain concepts, different language will have their unique corpus sets. Identifying the correspondence relationships between different lauguage corpus sets is a new direction to be explored in IFM Lab.
Knowledge and concepts in the real-world can be represented as a network structured diagram outlining their intrinsic relationships, namely the knowledge graphs. Many knowledge and concepts are shared by different knowledge graphs at the same time. Fusion of knowledge graphs from different sources is important for cross-source synergistic knowledge discovery.
In modern enterprise, to facilitate the communication among employees, a new type of online soical networks have been launched inside the firewall of the companies, namely the enterprise social networks (ESNs). Effective fusion of enterprise internal information sources, like ESNs, organizational chart, employee profile information and collaboration notes, for knowledge discovery is an important research projects in IFM Lab.
About the patients, different types of information will be collected before determining the desease they may have, including the brain fMRI image, regular physical diagnosis, and various drug tests. These complementary informaiton sources will provide a comprehensive information about the patient. Effectively fusing these information sources will lead to more precise diagnosis results for the patients.
From the government and traffic service providers, different kinds of data about cities can be obtained, like crime rate, population density, bus drop-off points, metro train routes. Effective fusion of such diverse information sources together provides a new research direction for studying traffic and urban planning problems from the data driven perspective.